AI-Powered Fraud Detection: How FinTech Companies Save Millions
This article explores how AI-powered fraud detection helps FinTech companies save millions by identifying fraudulent activities in real time. It details the AI implementation at XYZ FinTech, highlighting its success in reducing fraud, improving security, and enhancing customer trust.
CASE STUDY
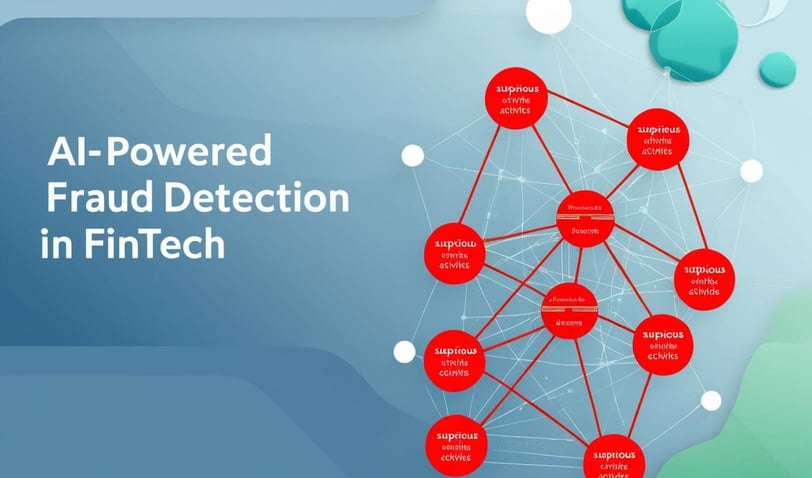
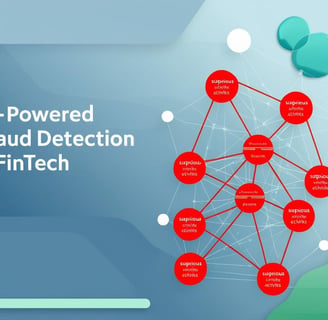
The financial technology (FinTech) industry is transforming the global financial landscape, making transactions faster, more accessible, and convenient. However, this growth also presents increased risks, with fraudsters exploiting digital systems. Enter artificial intelligence (AI): the game-changer in fraud detection. This case study delves into how FinTech companies leverage AI to save millions, offering insights into applications, challenges, and the future of AI-powered fraud prevention.
The Growing Threat of Financial Fraud
Digital transactions have soared in recent years, driven by innovations in mobile banking, peer-to-peer payment apps, and cryptocurrencies. Unfortunately, this growth has led to an alarming rise in fraudulent activities:
- Identity theft: Criminals steal personal data to impersonate individuals for financial gain.
- Transaction fraud: Unauthorized transactions occur due to compromised accounts or payment systems.
- Synthetic fraud: Fraudsters combine fake and real information to create synthetic identities for financial exploitation.
- Phishing attacks: Scammers deceive users into revealing sensitive data.
The need for robust fraud detection systems has never been greater. According to a recent report, financial fraud costs global businesses over $32 billion annually, making efficient fraud prevention a top priority for FinTech companies.
How AI Enhances Fraud Detection
AI-powered fraud detection systems leverage machine learning, natural language processing, and other AI techniques to identify and mitigate fraudulent activities. Unlike traditional rule-based systems, AI-driven solutions adapt to evolving fraud patterns, making them more effective and resilient.
Key Features of AI-Powered Fraud Detection
1. Real-Time Analysis
- AI systems monitor transactions in real time, flagging suspicious activities instantly.
- Example: AI identifies anomalies in transaction patterns, such as unusually high spending from a user’s account in a foreign location.
2. Behavioral Analysis
- AI profiles user behaviors, detecting deviations that may indicate fraud.
- Example: A sudden change in login behavior, such as accessing an account from an unrecognized device, triggers a security alert.
3. Predictive Analytics
- Machine learning models predict potential fraud by analyzing historical data.
- Example: AI predicts which accounts are at higher risk based on past fraud incidents.
4. Natural Language Processing (NLP)
- NLP tools analyze phishing emails or messages to identify fraudulent communications.
- Example: AI scans emails for suspicious language patterns, helping users avoid phishing scams.
5. Advanced Data Integration
- AI integrates data from multiple sources, such as payment systems, social media, and customer databases, to build comprehensive fraud profiles.
- Example: Cross-referencing credit card usage data with geolocation to detect inconsistencies.
Case Study: FinTech Leader Leveraging AI for Fraud Detection
Background
XYZ FinTech, a global leader in digital payments, faced mounting losses due to fraud. With over 50 million users worldwide, the company needed a scalable, efficient solution to mitigate risks and protect customers. Traditional rule-based fraud detection systems proved inadequate, as fraudsters evolved their tactics faster than rules could be updated.
Implementation of AI
To address this challenge, XYZ FinTech partnered with an AI solutions provider specializing in fraud detection. The implementation followed these steps:
1. Data Collection
- Historical transaction data, user profiles, and previous fraud incidents were aggregated for training AI models.
- Over 10 terabytes of data were processed, ensuring comprehensive model training.
2. Model Development
- Machine learning models were developed to detect fraud patterns.
- Techniques such as neural networks and decision trees were used to improve accuracy.
3. Real-Time Monitoring
- AI systems were integrated into the company’s transaction processing pipeline, enabling real-time fraud detection.
4. Continuous Learning
- The system continuously updated its models based on new fraud incidents, improving detection capabilities over time.
Results
Within six months of implementation, XYZ FinTech reported:
- Fraud Reduction: A 75% decrease in fraudulent transactions, saving $12 million annually.
- Customer Satisfaction: Improved user trust due to enhanced security measures.
- Operational Efficiency: Reduced manual fraud investigations by 60%, allowing staff to focus on high-value tasks.
- Regulatory Compliance: The AI system ensured compliance with anti-fraud regulations, avoiding hefty fines.
Benefits of AI-Powered Fraud Detection
1. Scalability
- AI systems handle vast amounts of data efficiently, making them ideal for global FinTech companies.
2. Accuracy
- Machine learning models reduce false positives, minimizing disruptions for legitimate users.
3. Cost Savings
- Automating fraud detection saves millions in potential losses and operational costs.
4. Proactive Approach
- AI anticipates fraud before it occurs, unlike traditional reactive systems.
Challenges in AI-Powered Fraud Detection
While AI is transformative, its implementation comes with challenges:
1. Data Quality and Availability
- AI models require vast amounts of high-quality data, which may be difficult to obtain.
- Solution: Invest in robust data collection and cleaning processes.
2. Evolving Fraud Tactics
- Fraudsters continuously adapt their methods, challenging AI models.
- Solution: Implement continuous learning mechanisms to update AI systems.
3. Privacy Concerns
- Collecting and analyzing user data raises privacy issues.
- Solution: Adhere to data protection regulations such as GDPR and CCPA.
4. High Implementation Costs
- Initial AI deployment can be expensive for smaller firms.
- Solution: Leverage cloud-based AI solutions to reduce costs.
Future of AI in Fraud Detection
AI’s role in fraud detection will expand as technologies evolve. Key trends include:
1. Explainable AI (XAI)
- XAI will enhance transparency, allowing companies to understand AI decisions and improve trust.
2. Blockchain Integration
- Combining AI with blockchain will create tamper-proof transaction records, reducing fraud opportunities.
3. Edge Computing
- Deploying AI at the edge will enable faster fraud detection, even in low-latency environments.
4. Collaborative AI Networks
- FinTech companies will share anonymized data through AI networks to strengthen fraud prevention collectively.
Conclusion
AI-powered fraud detection is revolutionizing the FinTech industry, saving millions annually and safeguarding customer trust. By leveraging advanced machine learning algorithms, real-time analytics, and continuous learning, FinTech companies like XYZ FinTech are not only combating fraud effectively but also setting new benchmarks for security and efficiency. As AI technologies advance, the fight against financial fraud will become even more robust, ensuring a safer digital financial ecosystem for all.
By embracing AI in fraud detection, FinTech companies can stay ahead of fraudsters, protect their bottom lines, and enhance customer confidence. The journey of XYZ FinTech serves as a compelling example of how AI transforms challenges into opportunities, shaping the future of financial security.
Read more relevant articles at :"AI in Agriculture: Enhancing Crop Yield with Predictive Analytics".
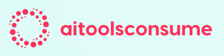
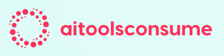